Updated April 19, 2024: As AI and predictive modeling marketing in particular continue to develop, we’ve refreshed the article to reflect the latest advantages of integrating them into your sales workflow.
Customer data has always been essential to high-leverage decision-making. The more we know, the better decisions we can make. But while the data of the past was easy to keep in one’s head, today we generate and track millions of data points that require powerful analytical tools to process.
In the digitization era, companies are used to engaging with and buying products online. Even significant enterprise software contracts might start with a quick message in an online chat.
That’s why online marketing campaigns and advertising are becoming more sophisticated and consequential to business growth.
Data is king.
If you have more accurate data than your competitors, you’ll always be a few steps ahead, targeting the market with more precision while spending less.
This creates a cycle of ever-improving data analysis and allows you to leverage predictive modeling marketing, which can improve your conversion rates and bring in more revenue.
Tip: Get the best of predictive capabilities in one automated tool with Lift AI. It integrates right into your marketing stack and identifies website visitors with the highest buyer intent, simplifying your sales process.
What Is Predictive Modeling and How Can it Benefit Marketing Teams?
Predictive modeling in marketing allows companies to find the most effective marketing strategy and make better marketing decisions by leveraging data with the help of AI or machine learning.
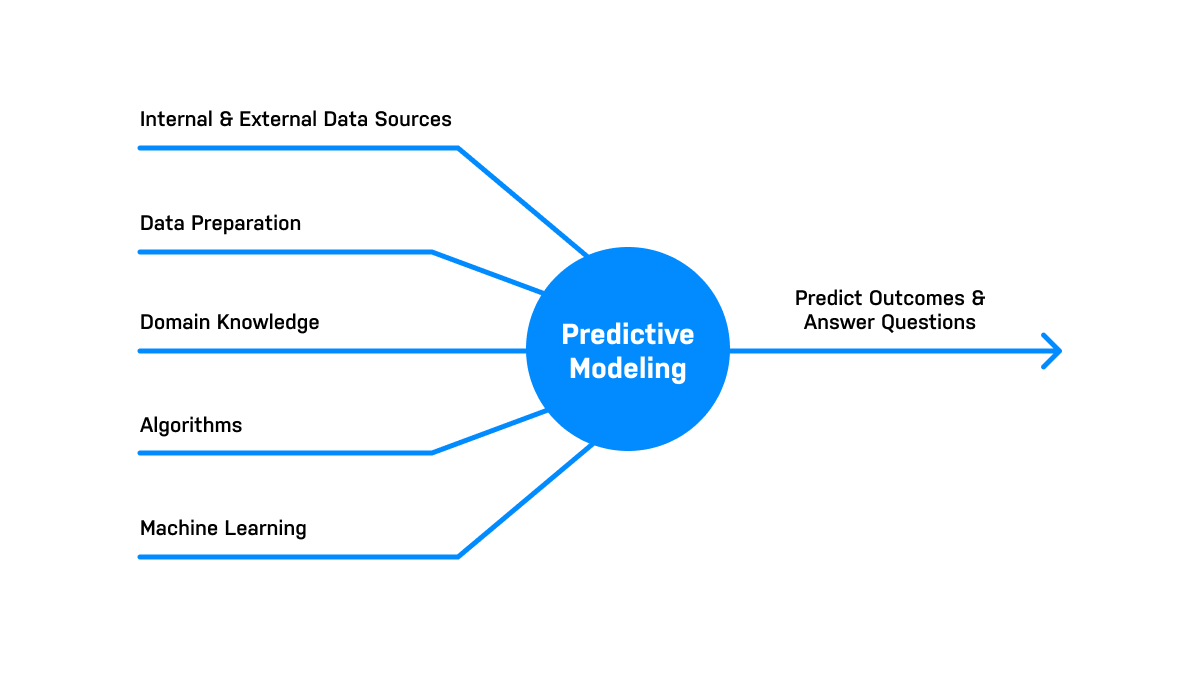
(Source)
Predictive modeling is a statistical technique that analyzes historical data to predict future outcomes, building a mathematical model to identify relationships and trends in the data and predict outcomes based on these insights.
There are two types of predictive modeling marketing:
- Autonomous (e.g. showing tailored product recommendations based on past behavior)
- Informative (e.g. suggesting a better headline for a newsletter)
Both types evaluate millions of data points, from time to location to demographics to past actions, to produce accurate results.
A few years ago, AI capabilities on this scale were only available to leading tech giants with in-house data scientists. Today, predictive analytics and modeling can be used by any company that sees the benefit of leveraging the latest technology to reach its goals.
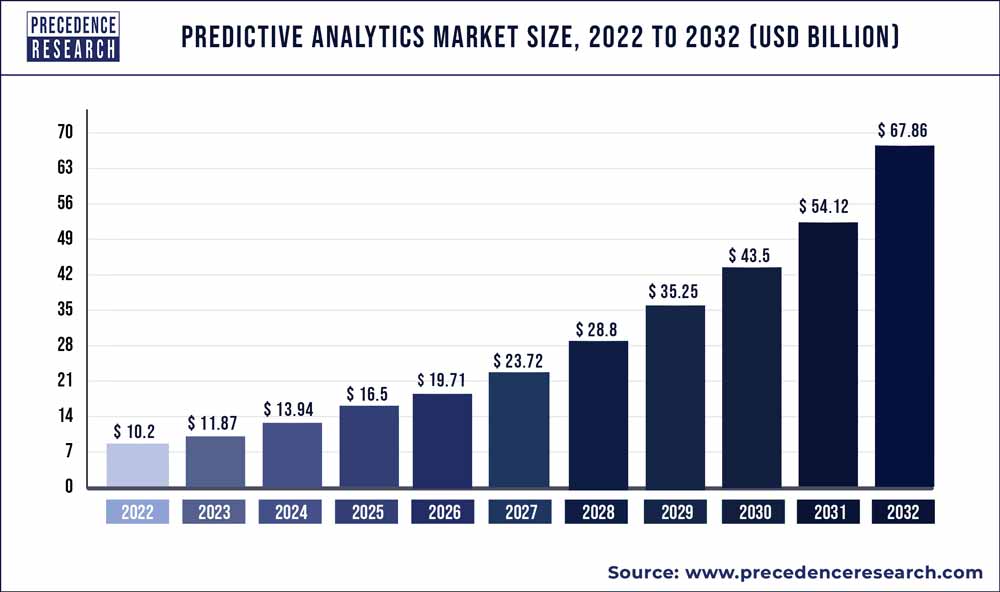
And we've still only begun to scratch the surface of potential uses for predictive analytics and modeling. A recent Precedence Research report projects that the predictive analytics market will reach $67.86 billion by 2032.
Types of Predictive Models Used in Marketing
There are several common types of predictive models marketers use to forecast customer behavior and optimize campaigns:
Clustering models
This predictive model is used to segment customers into groups based on shared characteristics or behaviors. You can customize your marketing messages and campaigns by strategically identifying customer segments to maximize their impact and drive results.
HubSpot identifies seven different types of segmentation models that companies can use to easily personalize customer experiences.
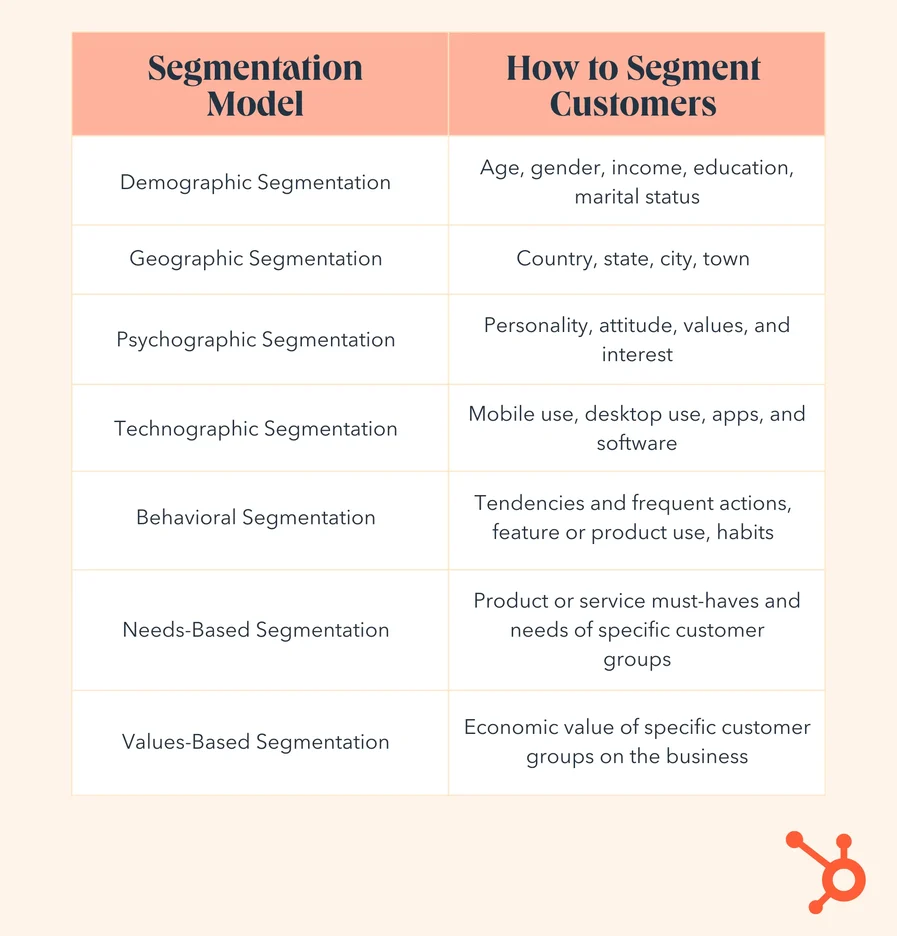
For example, a clothing retailer could use a clustering model to pinpoint customers who prefer high-end jeans and target them with email campaigns promoting their new designer line.
Propensity models
Also called predictive scoring, propensity models forecast the probability of a customer engaging in a specific action, like purchasing a product, canceling a service (churning), or responding to a marketing campaign. These models analyze past customer behavior and pinpoint patterns marketers can use to predict future behavior.
Lift AI, for example, uses machine learning and behavioral data to assign a buyer intent score to every website visitor, whether they're known or anonymous. This predictive modeling technique allows companies to create custom engagement strategies that more closely align with visitors' intent.
Here, we see how a company may use chat technology to provide a targeted customer experience while also increasing the efficiency of its sales team's efforts.
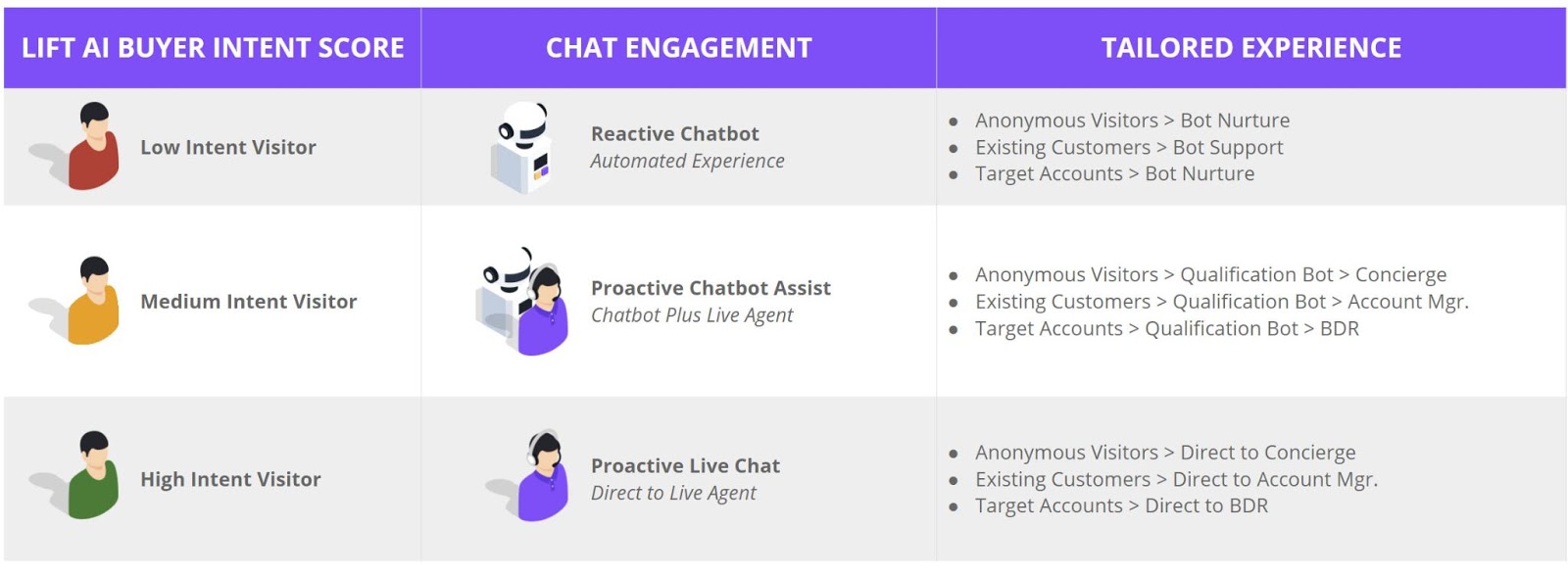
Propensity models can also be used to improve customer retention and reduce customer churn. This predictive model is especially valuable for subscription services that want to pinpoint customers who are at risk of canceling their subscriptions so they can provide them with a compelling discount or other incentive to ensure they remain loyal.
Collaborative filtering models
Collaborative filtering models are a powerful tool for recommending products and services based on customer behavior and similar customer preferences.
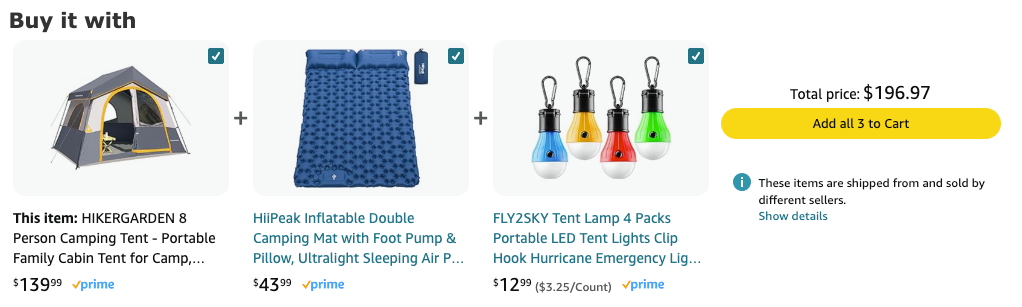
There are two types of collaborative filtering:
- User-based measures similarities between users. For instance, a streaming service could use collaborative filtering to offer personalized movie recommendations by analyzing a customer's previous movie choices and those of similar users with comparable tastes.
- Item-based measures similarities between items that users rate or buy, and other items. Amazon uses this extensively to upsell additional items that can accompany what a user is browsing to purchase.
In addition to Amazon, this predictive model powers the recommendation engines used by companies like Netflix, LinkedIn and Spotify.
Regression models
Regression models are statistical techniques that uncover the relationship between one or more independent variables (factors you can influence) and a dependent variable (the outcome you want to predict). This translates to understanding how different factors impact key metrics.
Marketing and sales teams can use regression models to:
- Optimize marketing campaigns
- Perform price sensitivity analysis
- Predict customer lifetime value (CLV)
- Optimize sales territories
- Forecast sales
- Measure marketing ROI
- Find opportunities to upsell and cross-sell
Time series models
This predictive model forecasts future events based on trends in historical data. It's especially helpful for predicting factors like seasonal sales fluctuations, inventory management, website traffic patterns, or product demand.
As this graphic from Intelliarts shows, time series modeling is an effective tool across many industries and use cases outside of sales and marketing.
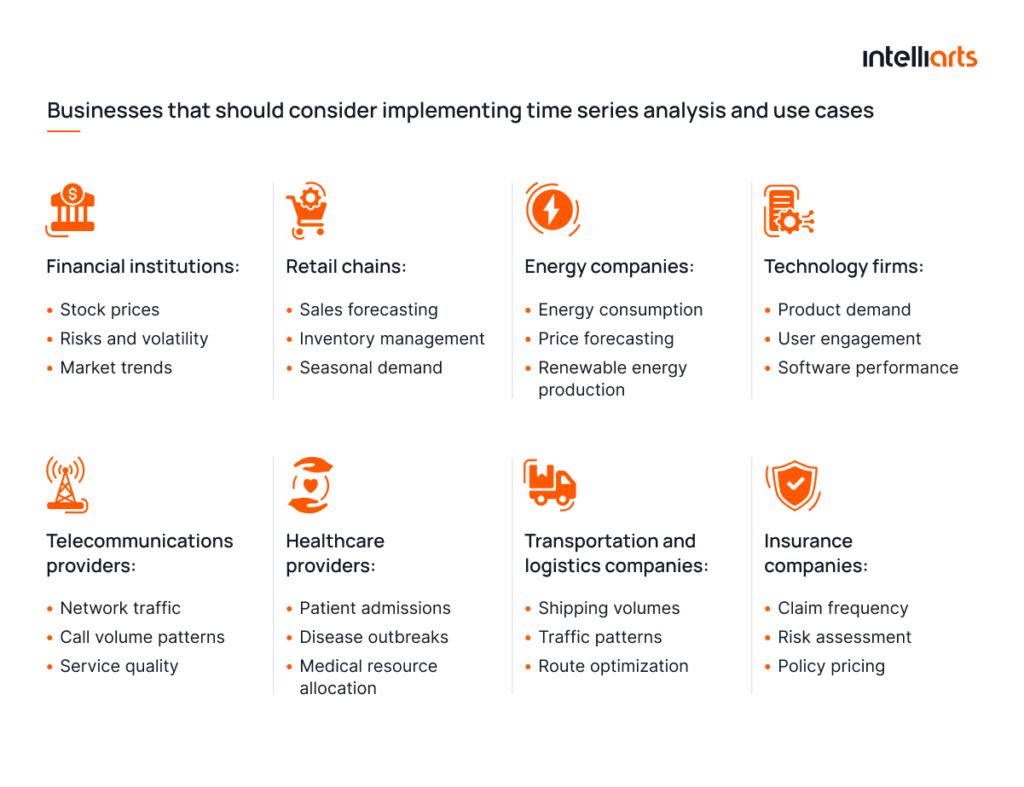
By understanding these cyclical trends, marketers can better plan resource allocation and optimize marketing campaigns and pricing strategies.
Why You Should Add Predictive Modeling to Your Marketing Strategy
Predictive modeling can benefit your marketing efforts in multiple ways, from providing new insights to automating tasks to increasing revenue.
Unprecedented Insights
Predictive models help you better understand your customers’ behavior by utilizing historical data. This data includes past actions of either your actual customers or a similar cohort of visitors for their analysis.
As a result, you can take the guesswork out of your plans and adjust your strategy in a way that improves the efficacy of your marketing campaigns, lead generation, and conversion opportunities.
Task Automation
Predictive tools can help automate your sales and marketing activities. For example, rating the buyer intent of various website visitors allows your marketing team to focus on those who are a better fit for your product or service instead of trying to sell to everyone.
Revenue Lift
Predictive modeling applications can predict customer behavior to improve your marketing channel mix, identify upselling or cross-selling opportunities, segment customers, increase retention, and guide customer acquisition. All of these lead to better revenue growth.
Asset Optimization
Predictive models rely on visitor interactions with your digital assets like your website, and that data can become invaluable in optimizing performance.
For example, predictive tools can track the performance of your online ads, website user experience, and calls to action. You can then use this data to write better headlines, adjust targeting, or improve UX design. Lots of SEO and martech apps are already offering such capabilities.
It's useful to think of predictive modeling marketing in terms of cost per lead. If an average B2B lead costs you between $50 and $100, acquiring a single customer at 1% conversion could set you back $10,000.
With predictive models, doubling your conversion rate doesn't take too much effort, but it saves you thousands of dollars on every customer and allows you to reinvest into generating even more conversions for the same spend.
That's why predictive modeling in marketing is growing rapidly right now.
Predictive Modeling Marketing Challenges
While predictive modeling offers significant benefits, marketers should be aware of potential challenges in their marketing strategies:
Data Quality
Predictive models are only as good as the data they are built on. Ensuring data is accurate, complete, and representative of the target audience is crucial for reliable predictions.
Interpretability
Some advanced modeling techniques, like neural networks, can be difficult to interpret. Marketers may struggle to explain why the model made certain predictions or how to act on the insights.
Changing Conditions
Major events like the COVID-19 pandemic can dramatically alter customer behavior, making pre-existing models less accurate. Models need to be regularly updated to account for changing conditions.
Resources and Expertise
Building and deploying predictive models often requires specialized skills in data science and machine learning. Marketers may need to invest in training or partner with experts to fully leverage predictive analytics.
Despite these challenges, the competitive advantages of predictive modeling are clear. By understanding customer behavior, optimizing campaigns, and acting on data-driven insights, marketers can drive more conversions and revenue with predictive modeling.
How to Apply Predictive Modeling in Your Marketing Strategy
There’s a wide range of marketing activities where predictive marketing can be of help. One of the most effective ones is identifying buyer intent.
Unlike your BDRs, predictive models rely on all available past and real-time information to make decisions, including visitors’ demographics, behavior, and activity patterns. Combining thousands of variables, the models can then assign strong lead scores to visitors with high buyer intent.
In turn, marketers can use that knowledge to deploy the most effective tactics to engage each visitor cohort (from low to high buyer intent).
Lift AI is the leading buyer intent solution in the B2B market today. It uses a proprietary machine-learning model that incorporates 15 years of sales insights and more than one billion data points to identify visitors with the highest buyer intent on your website, even if they are anonymous (as up to 98% on a typical website could be).

This is how Lift AI assesses buyer intent and provides predictive lead scoring:
- Visitors come to your website through various marketing channels
- Lift AI scans each visitor to determine their buyer intent (with 85% accuracy) by evaluating their real-time behavior
- Visitors who get a high score can be directly connected to your sales team (e.g. through a chat platform) to get the best chance of conversion
- Visitors with medium and low scores can be greeted with a nurturing bot or a self-help guide to be efficiently moved to the next step of the conversion funnel
On average, 9% of your traffic has high buyer intent. Connecting them to your salespeople in the most efficient way can significantly improve your website performance.
Drive Increased Pipeline and Sales Efficiency With Lift AI
Companies using Lift AI have reported that within 90 days their chat conversions increased anywhere from two to 10 times.
PointClickCare increased conversions by 400%, adding over $1M of incremental revenue in the first year. Formstack, another Lift AI customer, increased pipeline sales by 420%.
Predictive marketing works because it leverages new technologies to improve well-established sales patterns.
Integrate predictive marketing into your workflow today by getting in touch with us.
Signing up is as easy as adding a JavaScript snippet to your website. After that, Lift AI can be automatically integrated with your marketing stack, from chat platforms to CRMs. There’s nothing better than seeing the results of predictive tools for yourself